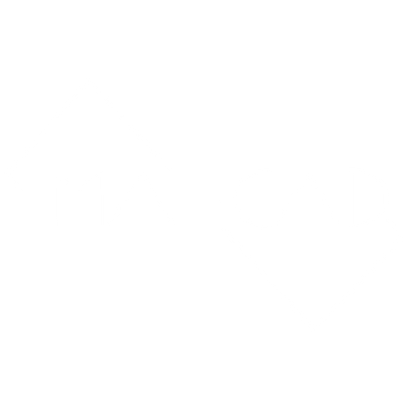
Transforming raw DATA into insightful and actionable INFORMATION to obtain KNOWLEDGE
News & Interests
just in:
6 December 2024 - WMTC 2024, Chennai, India
We are happy to share the presentation given at WMTC 2024 for your peruse:
4-6 December 2024 - WMTC 2024, Chennai, India
We are thrilled to announce that we will be presenting our paper, "Vessel Data Visions," at the World Maritime Technology Conference 2024, held on 4-5 December in Chennai, India.
This groundbreaking paper explores the integration of the Ship Digital Twin with advanced technologies such as knowledge graph databases and deep learning, aiming to revolutionize the operational and maintenance efficiencies of maritime vessels.
At the heart of this innovation are "vessel visions"—customized data views designed to meet the unique needs of diverse maritime stakeholders, including class societies, flag states, ship operators, port authorities, and more. Each vision is developed using a domain-specific language (DSL), enabling tailored interfaces that provide precise access to and interpretation of the digital twin’s data.
Join us in Chennai to dive into the future of maritime technology and discover how the Ship Digital Twin is shaping the industry!
Stay tuned for updates and insights from WMTC 2024!
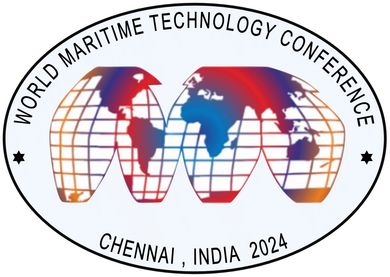
4 July 2024 - Life Cycle Wide Data Visions
In the rapidly evolving field of engineering, the importance of data governance across the entire lifecycle of a product cannot be overstated. The keynote presentation, "Life Cycle Wide Data Visions," delves into the transformative impact of digital twins on data management from conceptual design to decommissioning. As products progress through various stages—conceptual design, detailed design, manufacturing, installation, operation, and finally, decommissioning—their digital twins evolve correspondingly, embodying unique 'Data Visions' at each phase. These visions are not static but dynamic, adapting and integrating the myriad of external data streams such as environmental conditions, geographical changes, and operational contexts. This presentation explores how these sophisticated digital twins, through their continuous transformation and interaction with external data, serve as crucial decision-making tools.
Please, click the button below to download slides from the presentation.
9 February 2024 - AI in Ship Life Cycle
The lecture titled "AI in Ship Lifecycle" for the Indian Maritime University on February 9, 2024, will explore the innovative application of Artificial Intelligence (AI) in managing the life cycle of ships. This presentation focuses on leveraging AI to enhance decision-making in ship design, operation, and maintenance. It includes an in-depth look at developing a digital twin of a ship, utilizing a knowledge graph database for semantic information storage, and employing deep learning for advanced decision-making. The session will also cover the evolution and impact of the MA-CAD method, emphasizing its role in minimizing costs and risks in maritime operations.
2023/2024 - Season's Greetings
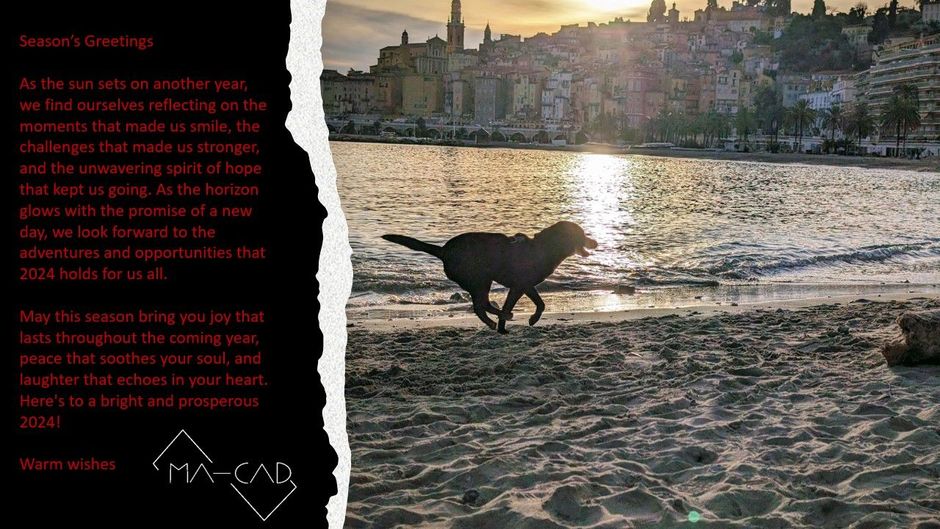
19 December 2023 - Vessel Vision project presented to FERIT students (Osijek, Croatia), envisioning creation of student assignments in line with project goals.
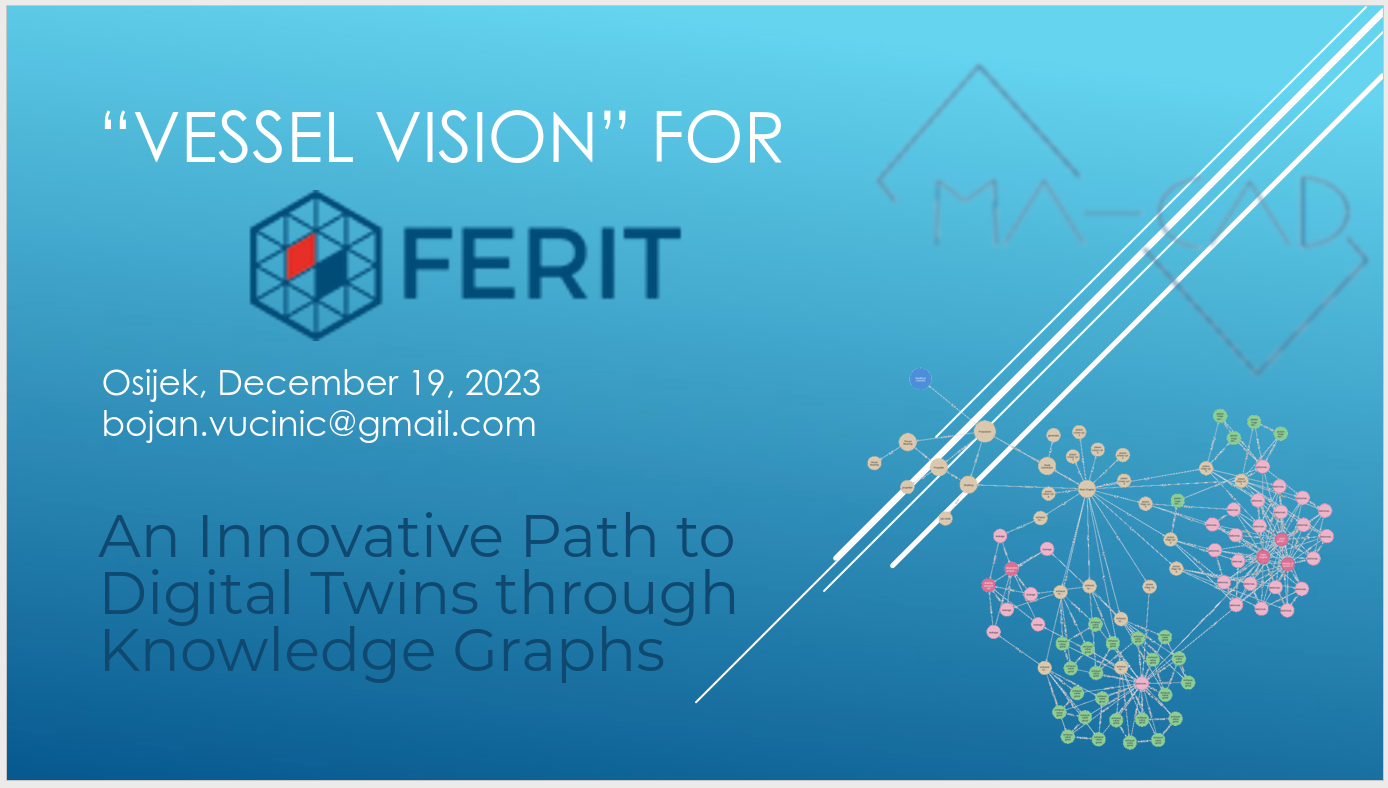
5 September 2023 - neo4j platform selected for Vessel Vision project
The neo4j platform has been selected to home the digital twin knowledge graph.
18 August 2023 - Vessel Vision project unveiled
Thrilled to unveil our latest endeavor: the "Vessel Vision" project. Anchored in innovative technology and forward-thinking, this initiative promises to redefine how ships interact with our world, both above and below the waves.
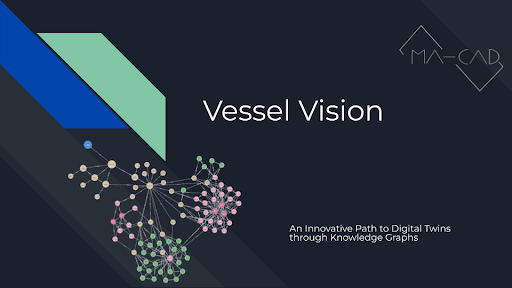
13 July 2023 - Life Cycle Costs Calculator ported to Flutter/Dart
Exciting news! MA-CAD's Life Cycle Costs Calculator, now in its 3rd version, has been ported to Flutter/Dart. This transformation opens up new horizons, allowing the software to run seamlessly on various platforms. Whether you're using Android, iOS, Linux, or Windows, or simply browsing the web, this updated version ensures consistent performance across all platforms. The same code base, multiple functionalities - an unparalleled convenience in the realm of cost calculation!
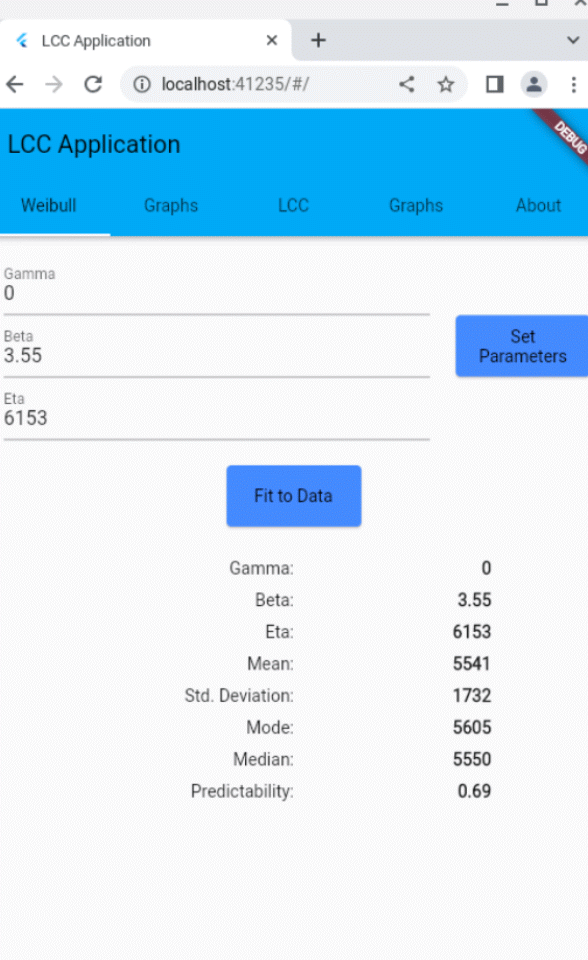
23 March 2023 -
Failure Knowledge Graphs - published on OnePetro
8 March 2023 -
Failure Knowledge Graphs - presented at SOME 2023
January 2023 - Season's Greetings
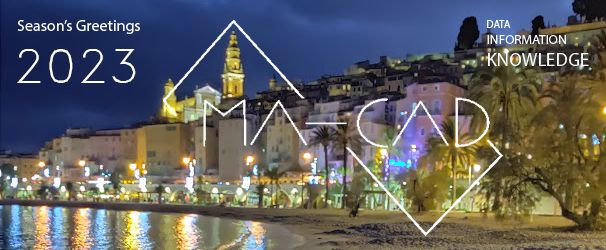
July 2022 - MA-CAD website back online. Thanks for your patience!
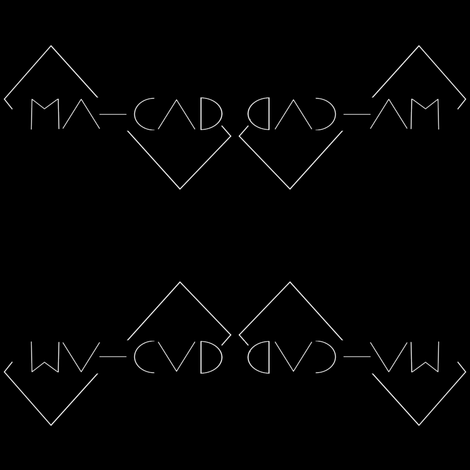
May 2021 - The (R)Evolution of Risk
The definition of risk (at least in technical applications) has always been a probabilistics one...
Projects
This section introduces you to some of our work through a selection of our projects:
Vessel Vision
At the heart of the 'Vessel Vision' project lies the concept of a 'Digital Twin'. For those unacquainted, think of it as a mirror image of a real-life ship but in a digital realm. This isn’t just a static representation but an evolving, data-driven model that keeps pace with its physical counterpart in real-time.
The neo4j graph database platform has been selected as a persistent store.
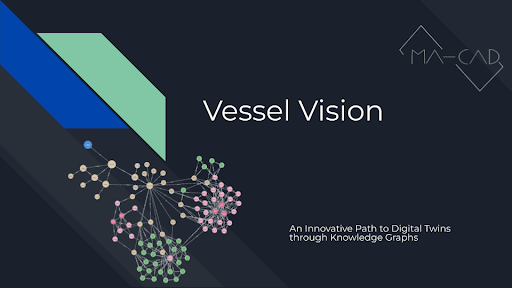
Life Cycle Costs Calculator
Life Cycle Costs Calculator the MA-CAD software has been ported to Flutter / Dart. This is version 3 of the software. The port means that for the same code base the application can run on mobile (Android, iOS), desktop (Linux, Windows), and in the web browser.
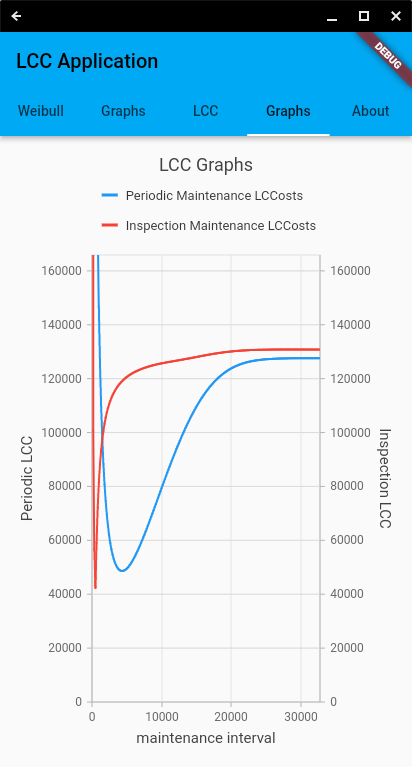
Failure Knowledge Graphs
Failure analysis is the cornerstone of asset management via life-cycle costs optimizations. Knowledge graphs are semantic nets that are the next level of database technology. Deep learning is a field of inquiry devoted to understanding and building methods that “learn”, that is, methods that leverage data to improve performance on some set of tasks. We propose to structure the deep learning data into knowledge graphs to foster advanced failure analysis leveraging optimum life-cycle costs.
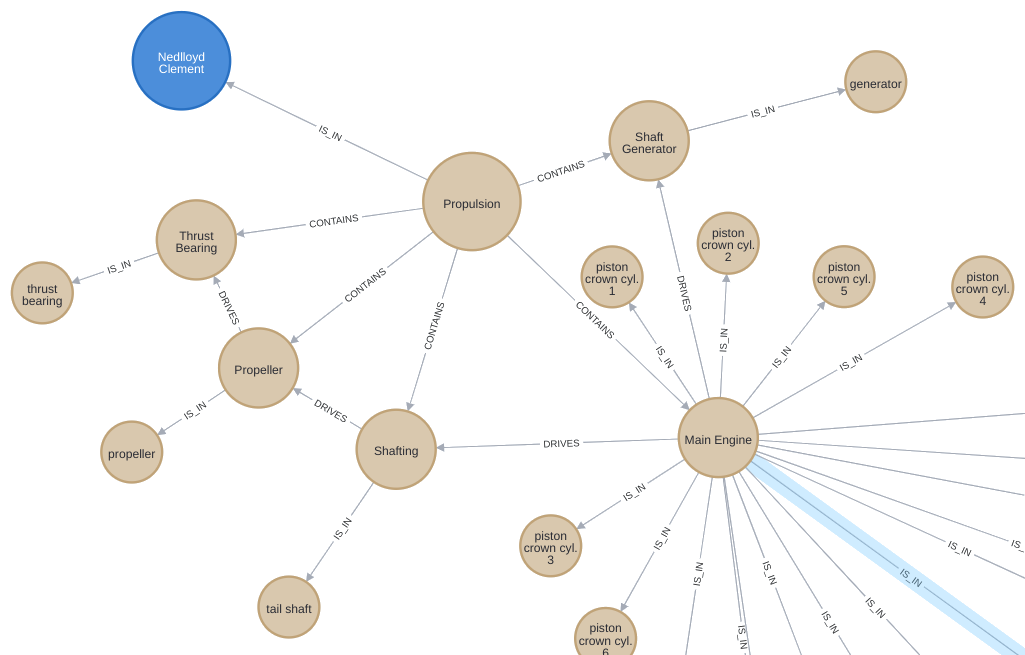
Towards a United Nations Environmental Airspace Agency UNEAA
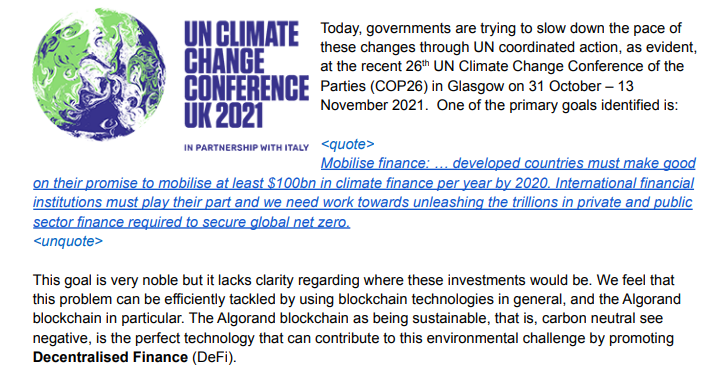
Digitalisation of the MA-CAD method
The “MAintenance Concept Adjustment and Design” is a book (ISBN 90-370-0112-2) published in 1994. This states the methodology applied to marine engineering systems of sea-going vessels. The project was sponsored by Nedlloyd Lines (today part of Maersk) and the Royal Dutch Navy.
The document will be digitized and re-published.
Open-sourcing of the MA-CAD Life-Cycle Costs Optimizer
The method includes a software tool with a Weibull data-fitting method and cost optimization methods for different maintenance policies.
The software will be open sourced and published on github.
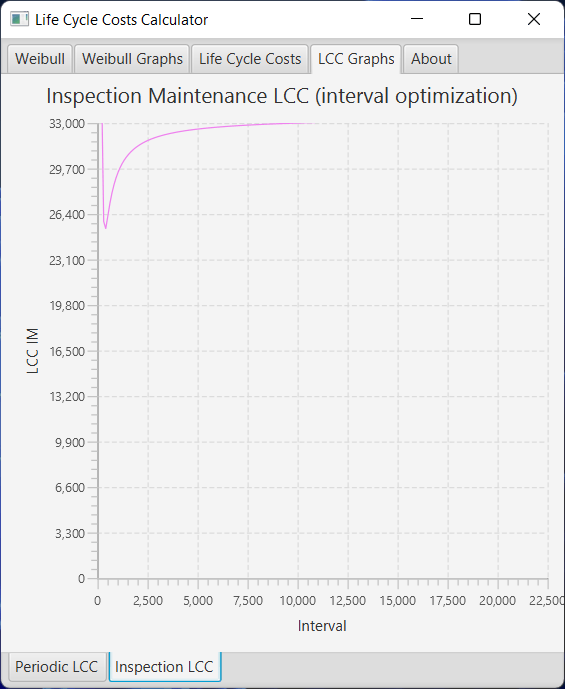